By Clint Boulton
Many IT leaders will tell you hiring tech talent is right up there with culture change as a chief hurdle to business transformation. Finding enough software engineers, Scrum masters, DevOps leaders and other potential change agents remains a burden. But experts agree the top challenge is hiring experts in data science, including those with machine learning (ML) and artificial intelligence skills.
From healthcare to financial services, every sector is embracing some form of AI as a core business strategy. Eight-four percent of 500 business leaders surveyed online by consultancy EY in 2019 said that AI is critical in facilitating efficiencies and reducing costs, gaining a better understanding of customers and generating new revenues.
But the road to success depends heavily on the talent pool, as 31 percent of those same leaders said that a lack of skilled staff is the No. 1 barrier to AI adoption.
Here experts share their experiences with mining AI talent and provide tips for how CIOs can lure the right mix of data scientists, ML engineers and AI experts.
Casting a wide net
Alan Jacobson was painfully aware of the dearth of data science talent when he joined Alteryx as chief data and analytics officer in April. Jacobson, who previously spent 25 years in engineering and other roles at Ford Motor, says that identifying the right talent to solve your business problems remains one of the toughest organizational challenges today. That rings true even for Alteryx, whose software makes it easier for citizen data scientists to visualize information for insights.
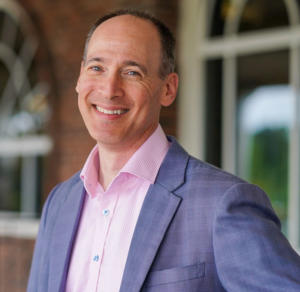
Alteryx
“Recruiting talent is hugely difficult,” Jacobson says. “There is more demand than supply.” One of the reasons for the difficulty is that candidates for data science, while likely possessing crack problem-solving skills, often hail from all manner of backgrounds and disciplines, says Jacobson, who was managing a team of 1,000 data scientists when he departed Ford as director of global analytics.
One of Jacobson’s top data scientists at Ford was a seismologist by training who graduated from MIT and taught herself SQL, Python, R and other programming languages. Others hail from agriculture, oceanography, political science and other domains that may seem unlikely for cultivating AI tools. “In data science, they can have almost any degree under the sun,” Jacobson says. “It’s more difficult than in many other professions to identify where people are on the data science continuum.”
Jacobson offers a couple of tips for zeroing in on data science talent:
Widen your aperture. A good data scientist combines interpersonal skills with an analytical mind. Recommended by a trusted friend, Jacobson’s seismologist cultivated soft skills while serving in the Peace Corps and teaching at a charter school. “Many of these experiences made her a phenomenal data scientist,” he says.
Identify problem solvers. Has a candidate solved real-world problems? Has a prospective hire built a software product or published an open source software library others use to solve problems? Perhaps they’ve competed in a coding challenge on Kaggle or other competitive platforms. These are key markers of whether someone may be a good fit for your team.
No data scientist stone left unturned
Identifying engineering talent who can build AI capabilities presents a more narrow challenge, often requiring coding skills, working with Bayesian statistics and experience developing algorithms.
With such a limited talent supply, the average AI job is posted for over 100 days, compared to software developer role, at less than 70 days, according to a Gartner talent acquisition published in early 2019. The researcher recommends broadening talent outreach beyond fertile grounds such as San Francisco Bay Area, New York City and Seattle to Austin, Philadelphia and Denver. Looking at these alternative locations will help organizations capture the market early and ease pressure on salary costs, Gartner says.
EY searches for AI engineers and data scientists from tech titans in Silicon Valley and rising startups all over the world, according to Jeff Wong, EY’s chief innovation officer. Where it can’t hire talent, it taps students of all problem-solving disciplines from universities worldwide. It also conducts significant cross-training and reskilling of staff across several disciplines, including topology, cryptography, physics and astrophysics.
Although 18,000 of its 270,000 global employees are data professionals, Wong believes that EY must do more to groom AI talent to help the consultancy reach new heights over the next decade.
“We’ve had very good luck, but our ambition outpaces our ability to recruit AI folks,” Wong tells CIO.com, adding that EY has doubled its core AI team every four months for the past several years. “Our ambition is to be the best in the world” for clients. Wong offered a few nuggets of advice for bagging AI talent.
It’s all about the data. Large data sets are a big draw for data experts looking to tackle big, hairy problems. EY, for example, is building AI software that automatically scans billions of pages of legal and tax documents filled with structured and unstructured data, Wong says.
Think about what you want to do. CIOs are all on different data journeys, but it’s critical for IT leaders to figure out exactly what problem they wish to solve before they allocate the AI talent best suited to work on it, Wong says.
Boiling it down: Buy, build and borrow
Recruiting talent requires a combination of approaches: Buy, borrow and build, followed by execution of projects that create a flywheel of continuous innovation, according to Mike Pino, a partner with PwC. What approach you choose depends on your corporate culture.
Buy. Hire AI staff where you find them, but be prepared to pay a premium, especially if you’re based in certain geographies. If you’re a CIO based in Milwaukee, Wisconsin, or Columbus, Ohio, you’re going to have a harder time filling positions than a peer in San Francisco, New York or even Austin, Texas, Pino says.
Borrow. Tap talent from consultancies or universities to work on projects or build products can help you fill short-term gaps in areas that “you don’t need to own,” Pino says.
Build. You can also groom talent in-house. This will take time and patience, but you can start slowly with the hope of letting talent bloom. PwC upskilled a small portion of its staff, including those of an entrepreneurial persuasion, to work on robotic process automation (RPA), and is making a similar push in AI and ML.
Once you have the talent you can identify champions to help construct AI solutions. Then it’s a matter of executing on those projects, monitoring progress, soliciting feedback and correcting course. Every business problem will require you to tweak your methods. “There isn’t a one-size-fits-all approach,” Pino says.
Source: CIO Magazine